As I sit here writing this, today is Friday 13th December, 2019 – an ominous and somewhat fitting date given the outcome of yesterday’s general election in the UK (depending on your political stance, of course). Although a week will have passed by the time this is posted, I feel that there is an increased onus on me to try and lift your spirits, perhaps by distracting you with some more light-hearted observations about Christmas TV. At the same time, I feel obliged to take this opportunity to reflect upon television’s role in relation to politics and the public sphere, particularly in the wake of the general election. As you can probably deduce from the title of this post, I’m afraid that I’ve opted for the latter.
As you read this, the 2019 general election will be old news. Well, at least a week old, which is a long time in politics. However, as I began the process of writing this blog, I wasn’t yet privy to the result. If I had used my various social media feeds as a barometer of who was likely to come out on top, then it would have been a one-horse race. But I’ve been here before and I’ve (eventually) learned my lesson.
In the lead up to the Brexit referendum in 2016 my Twitter timeline was filled with posts listing the numerous cultural, economic and political reasons as to why leaving the European Union was such a disastrous idea. It was equally filled with posts highlighting the various underhanded tactics of the leave campaign. People couldn’t possibly vote to leave based on all of this (mis)information, right? Wrong. Fast forward several months to the US presidential elections, and I still hadn’t quite learned my lesson. My feed was once again insisting that the prospect of Trump’s election was firmly within the realms of fantasy. I saw endless, optimistic tweets from Democratic Party supporters but there were few if any dissenting voices from the right. I felt betrayed when I saw the final result but soon realised that this misconception was largely my own doing. I choose to follow people with like-minded political views, and to mute or unfollow those who don’t, and in doing so I created my own algorithmic echo chamber. Research has shown that this is an experience that many of us have because the left and the right tend to be heavily isolated from one another within the virtual space of the Twittersphere (Brady et al., 2017).[i]
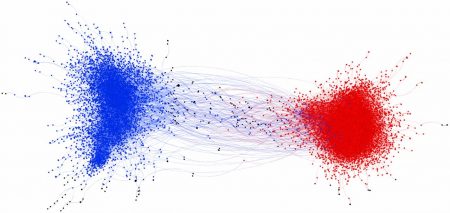
Fig 1. A visualisation of the left and right divide on Twitter. Source: https://pnas.org/content/114/28/7313
This phenomenon of the algorithmic echo chamber – also known as the “filter bubble” – is most visible in the realm of social media. This is because these platforms were built with personalization in mind. It is their raison d’être. From this perspective, filter bubbles can be viewed as a design feature rather than a design flaw because they help us to screen out and discard the vast amount of content that simply disinterests us. But the implications of filter bubbles are seismic. At best they can give us a false impression of reality, such as the outcome of an election. At worst they can interfere with the democratic process itself.
Filter bubbles are nothing new, but they are subject to increasing scrutiny in a world where much of, if not most of, the media we consume is delivered via a recommendation algorithm. The phenomenon of the filter bubble is also an increasingly pressing issue in the context of television, and particularly public service media [PSM], with many viewers (especially younger viewers) accessing content primarily or exclusively through algorithmically driven services such as the BBC iPlayer.
One way for a service such as the iPlayer to circumvent filter bubbles would be to implement a public service algorithm – something my colleague James Bennett first proposed in 2015 [ii] but which the BBC have only very recently begun to develop. To some extent, it’s understandable as to why a public service algorithm hasn’t been implemented sooner. For one thing, it was only as recently as 2017 (a whole ten years after the launch of the iPlayer) that they began to roll out a policy of requiring users to sign in. A key impetus for this move was so that the BBC would finally be able to design and deliver a public service algorithm built on user data.
Not long after the roll out their new sign in policy, senior BBC executives started bestowing the virtues of a public service algorithm. It began earlier this year in May with director of radio and education James Purnell who promised that the new BBC Sounds app would “pop your bubble” (qtd. in Savage, 2019), in reference to the filter bubbles we experience when using other music and radio apps such as Spotify. BBC Media Editor Amol Rajan spoke optimistically about Purnell’s plans in his analysis of the announcement:
An algorithm designed to promote scepticism rather than reinforce prejudice will not have the same commercial appeal as those that make, for instance, YouTube what it is. But, depending on its efficacy, it could potentially have a public benefit: Namely, to replace time-wasting with education (qtd. in Savage, 2019)
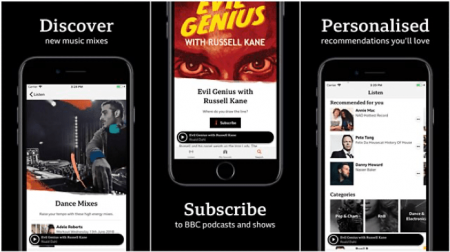
Fig 2. The new BBC Sounds app, where it seems that the BBC will be piloting their public service algorithm. Note the emphasis on personalisation.
Soon after Purnell announced his plans to burst our filter bubbles, director General of the BBC Lord Tony Hall pitched his idea for a “Netflix style algorithm for public service broadcasting” to peers on the House of Lords Communications Committee. Paradoxically, Lord Hall’s pitch relied on a comparison with an algorithm that is synonymous with filter bubbles. However, he explained to his peers that the BBC’s approach would differ: whilst Netflix uses viewer data to offer more of the same, the BBC’s plan is to use viewer data to ‘break the echo chamber of suggested content’ (Hall qtd. in Simpson, 2019) that is in many respects a selling-point of the iPlayer’s commercial rivals.
As promising as this all sounds, it all still feels rather vague. What exactly is a public service algorithm? What might it look like? How might it function? What might be its objectives? How might it be regulated and its efficacy be measured? To be fair, some of these questions have already been posed, though not yet answered, by those within the BBC (Fields et al., 2018) and by those further afield (e.g. Sørensen and Hutchinson, 2017).
Although these questions need to be answered in order to define and develop a successful public service algorithm, one can’t help but wonder why the BBC haven’t at least attempted to create one sooner, specifically one that doesn’t rely on user data [iii]. Surely it would be possible to develop an algorithm more in tune with the aims and objectives of PSM that is based on the metadata of the content (i.e. genre) rather than the data of the user – namely one that promotes a diverse range of programming based on the classification of content rather than a user’s past viewing habits.
A public service algorithm is a promising if not long-overdue development though in my view it might not go far enough. An algorithm that can recommend a wide variety of content is often envisioned as the ideal (and sometimes only) way to burst the filter bubble [iv]. Yet there has been little if any discussion as to how one might properly encourage or, dare I say it, enforce the actual consumption of the more varied content that such an algorithm would be designed to deliver. As such, I want to conclude this post with a number of somewhat radical and provocative suggestions. Some are more realistic than others, but all I hope will spark further debate about the nature and design of public service algorithms.
Firstly, and perhaps most provocatively, I propose that in addition to public service algorithms we might need to consider developing public service interfaces as well. Refining the process of content recommendation isn’t sufficient enough, it might also be necessary to change the process of interaction itself. For example, in order to ensure that the algorithm is effective the interface could limit viewer control, i.e. remove the opportunity to freely skip “challenging” or “diverse” content, or at the very least to require the viewer to watch a small amount of said content before they can move on to their next choice. In other words, putting a limitation on control might produce an experience more akin to the television schedule of yesteryear. If viewers can simply skip programmes to their heart’s desire, then what’s the point of a public service algorithm? Just watched three episodes of an American sitcom and want to watch another one? Too bad: here’s five-minutes of current affairs. Of course, I can’t imagine that this kind of proposal would go down too well with license fee payers or the general public, not least because it would ‘reopen the sticky question about PSB paternalism’ with many likely to view such a move ‘as forcing a ‘PSM diversity diet’’ upon iPlayer users (Sørensen and Hutchinson, 2017:97).
A second and somewhat less provocative idea, and one that is already being developed in the EU, would be to implement strict interface/content quotas. But instead of basing these quotas on the origins of content, it would be based on the perceived public service value of content. This would mean that a certain percentage of particular genres or programmes, the kind that Lord Hall and the BBC “thinks are good for us” (Hall qtd. in Simpson, 2019), are not only offered to viewers but are given due prominence in terms of their location within the interface. As you might expect, and as research demonstrates, content that doesn’t make it into the first couple of rows or columns of an interface receives far less attention, presumably resulting in fewer clicks. My own year-long analysis of the iPlayer reveals that the genres we might consider more aligned with the aims and objectives of PSM (current affairs, documentary, etc.) all feature far less prominently than those genres that are less often associated with public service values (comedy, entertainment, etc.)
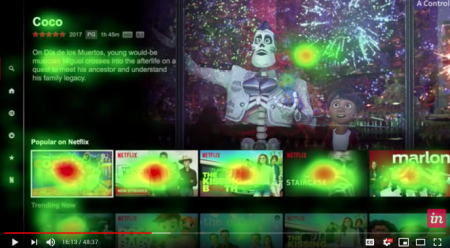
Fig 3: Research by Netflix that uses eye-tracking technology shows where viewers focus their attention within an interface. Source: Medium
Thirdly, if we can’t arrive at a consensus as to what a public service algorithm should do – should it promote a diverse range of content, should it embrace personalisation, should it somehow combine both, or should it do something else entirely? – then perhaps we should leave this choice in the hands of the viewer. In other words, the iPlayer could offer users control over the recommendation algorithm, giving them control over their own viewing experience. Those who are perfectly content with filter bubbles (which would probably be most) can ‘‘tune’ the level of personalization of the recommender system they are using to further boost personal autonomy’ (Helberger et al., 2016). Meanwhile, those who prefer the serendipitous nature of the television schedule can opt for a recommendation algorithm designed to burst their bubble.
Let me finish on an optimistic note. Although the election might not have turned out how many of us would have hoped, it has at least drawn our attention to the growing importance of “diversity exposure” (Helberger et al., 2016), a foundational public service ideal which some see as being under threat from commercial algorithms and the filter bubbles that they produce. And though the battle for the 2019 general election may be over, the pursuit of a public service algorithm – whatever that might be – has only just begun.
JP Kelly is a lecturer in film and television at Royal Holloway, University of London. He is the author of Time, Technology and Narrative Form in Contemporary US Television Drama (Palgrave, 2017). He has published essays in various books and journals including Ephemeral Media (BFI, 2011), Time in Television Narrative (Mississippi University Press, 2012) and Convergence. His current research explores a number of interrelated issues including narrative form in television, issues around digital memory and digital preservation, and the relationship between TV and “big data”.
References
Brady, W.J., Willis, J.A., Jost, J.T., Tucker, J.A and Van Bavel, J.J. (2017) ‘Emotion shapes the diffusion of moralized content in social networks’. Proceedings of the National Academy of Sciences. July 11, 114:28, 7317-7318. doi: 10.1073/pnas.1618923114.
Fields, B., Jones, R. and Cowlishaw, T. (2018) ‘The Case for Public Service Recommender Algorithms’. FATREC 2018 [conference]. Vancouver, Canada. Oct 6. Available at: https://piret.gitlab.io/fatrec2018/program/fatrec2018-fields.pdf.
Helberger, N., Karppien, K. & D’Acunto, L. (2016) ‘Exposure diversity as a design principle for recommender systems’. Information, Communication & Society. 21:2. 191-207. doi:10.1080/1369118X.2016.1271900.
Savage, M. (2019) ‘BBC building ‘public service algorithm’. BBC News. 13 May. Available at: https://bbc.co.uk/news/entertainment-arts-48252226.
Simpson, C. (2019) ‘Director-general wants Netflix-style algorithm for BBC’. Independent.ie. June 18. Available at: https://independent.ie/entertainment/directorgeneral-wants-netflixstyle-algorithm-for-bbc-38230987.html.
Sørensen and Hutchinson, J. (2017) ‘Algorithms and Public Service Media’ in Gregory Ferrell Lowe, Hilde Van den Bulck and Karen Donders (eds.) Public Service Media in the Networked Society. Gothenburg: Nordicom. 91-106. Available at: https://nordicom.gu.se/sv/publikationer/public-service-media-networked-society.
Notes:
[i] See also: https://theguardian.com/politics/2017/feb/04/twitter-accounts-really-are-echo-chambers-study-finds
[ii] See also: Bennett, J. (2017) ‘Public Service Algorithms’ in Des Freedman and Vana Goblot (eds.) A Future for Public Service Television. London: Goldsmiths Press. 111-120.
[iii] To be clear, the BBC does use a recommendation algorithm for the iPlayer, but by their own admission this is not a “public service algorithm” (see Purnell and Lord Hall’s comments above).
[iv] For example, see: Helberger, N., Karppien, K. and D’Acunto, L. (2016) ‘Exposure diversity as a design principle for recommender systems’. Information, Communication & Society. 21:2, 191-207.